This is the multi-page printable view of this section. Click here to print.
Clinics
1 - Infrastructure setup
Here you can find an overview of the infrastructure setup comprised of Local Developer Environment, Feature Cloud, and on-premise server at the clinic.
The developer use their local environment to build their machine learning applications and provide them to the user at the clinic via the feature cloud app store, allowing the user at the clinic to securely retrieve the machine learning application and run them on their data locally.
This setup ensure that data resides on the clinic system and infrastructure at all times.
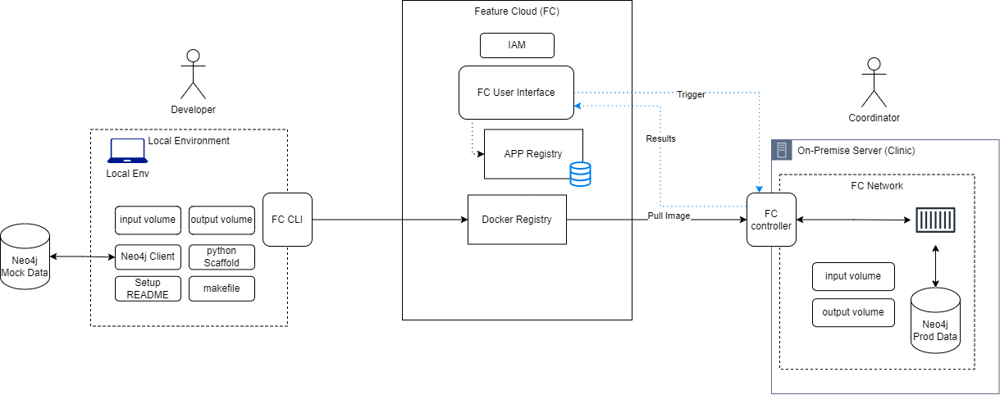
Developers obtain mock data from Neo4j in their local environment. The mock data mimics the data model on the clinic side.
Developers in the local environment follow the prepared template to build their machine learning applications and test them with the mock data. They can then upload their machine learning applications to the feature cloud app store.
The user at the clinic can select their machine learning application of interest from feature cloud app store and download them to their local environment via the pull process. They can then securely execute the machine learning application on their data in their local environment.
Depending on the performance of the application, the user at the clinic can choose to update the machine learning application residing on the feature cloud app store.
2 - About FeatureCloud
FeatureCloud is a lightweight pan-European AI development project aimed at mitigating cyber risks to healthcare infrastructure. Their focus is on creating innovative software toolkits employing federated learning with an all-in-one approach.
FeatureCloud addresses the main obstacles of AI and clinical data with two key characteristics: (1) no sensitive data is sent through any communication channels, and (2) data is not stored in one central point of attack.
More more information on FeatureCloud, please visit: https://featurecloud.eu/.
3 - Ensuring Compliance
The security and confidentiality of your data is our priority. For this reason, we use federated learning, a machine learning approach that does not require any data transfer to a centralized server or cloud. Instead, it allows the user to retrieve the model and run it on their data locally.
FeatureCloud provides an all-in-one federated learning platform and mediates the secure exchange and useability of the machine learning applications.
This ensures a complete separation of clinical data from the development environment and allows research partners from different clinics to collaborate without facing any data privacy restrictions or risking a central point of attack. Inference, results and predictions of the machine learning models used for diagnosis is in full control of the clinics and they can choose to update the global machine learning model as they see fit. If clinics decide to share their results with other entities, they must do so explicitly.
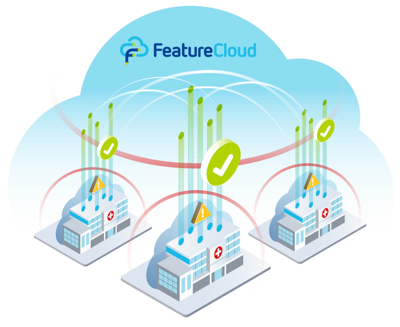